Data-driven Management for Safe and Reliable Railway Systems
As high-speed rail and metro systems are rapidly developing in speed and complexity in many cities and regions, identifying effective ways to ensure their safe, reliable and efficient operation has become more pertinent. A multi-disciplinary research project, led by CityU, has developed a platform of tools to improve safety and reliability in railway system health monitoring, crowd safety and disruption management.
“As with other large-scale technologies, there have been major accidents around the world related to high-speed rail and metro systems. These systems require continual improvement in condition monitoring, safety and reliability engineering, operation and maintenance, and decision systems to prevent accidents,” said Professor Tsui Kwok-leung, Chair Professor of Industrial Engineering and Adjunct Professor in the School of Data Science at CityU. He was the former project coordinator of the five-year Theme-based Research Scheme (TRS) project, with team members from CityU and other local and overseas institutions. The aim of the project was to innovate and advance rail system technologies to ensure safety, avoid and managing disruptions, and ensure operational efficiency.
“We are striving to fill at least two unique niches in high-speed rail and metro systems research,” said Professor Xie Min, Chair Professor in the School of Data Science and Department of Systems Engineering and Engineering Management (SEEM) at CityU, who is the current coordinator of the TRS project. “One is to develop new knowledge in self-cognisant fault detection and prognostics and health management of railway systems by synergising prominent sensor-based data management technologies and sophisticated modelling expertise. Another is to allow dynamic train deployment in response to abrupt events, accidents or disruptions using real-time transportation network data.”
Sensor-based monitoring of suspension systems
One of their key studies is the design of a novel domain-knowledge-guided data-driven framework to monitor and predict the health status of high-speed rail suspension systems by measuring real-time train vibration signals from sensors installed in multiple locations. “Suspension systems play a major role in high speed railways. The failure of springs and dampers may lead to accelerated wear of wheels and rails. Suspension damage may even increase derailment risk,” said Dr Li Lishuai, Assistant Professor in SEEM, who led the study.
Existing methods rely mainly on sophisticated dynamic models or simulations that require precise suspension and inertial parameter values. They are difficult to use in different rail systems, and the results can be distorted if the parameter values are inaccurate.
To overcome these limitations, the team proposed a model that can be trained quickly and adapted easily to different rail systems. It includes a feature extraction method, based on a simple dynamics model, to select the relevant information in the multi-location vibration data. Also, it introduces a novel way to generate training datasets via a simple dynamic model and impact analysis.
Having evaluated and tested the proposed method with 10 months of tracking data in a railway system operating in mainland China, as well as simulation data from different laboratories, the team found that it performed well. While more field tests are needed to prove its effectiveness and reliability, Dr Li believes the proposed method will be implemented in parallel with existing tools in the near future.
Analysing alighting and boarding behaviour
Applying an agent-based computer simulation model, Professor Lo Siu-ming, in the Department of Architecture and Civil Engineering, and his team conducted a study on passengers’ alighting and boarding movements in metro stations and the effects of passengers’ non-compliant behaviour. Different alighting and boarding rules in different passenger volume conditions were recommended to increase alighting and boarding efficiency.
Riding on the achievements of the TRS project, Professor Tsui said that several research projects with industry and university collaborators from Hong Kong, Taiwan and mainland China are ongoing, including establishing an escalator health condition analytics model, monitoring the wear of high-speed train wheels based on wheel profile data and multi-location vibration data, passenger flow forecasting for disruption management, traffic scheduling, and maintenance planning.
The research team expects the leading-edge technologies developed to not only contribute to safety and revenue management for inter- and intra-city rail systems, but also serve as a prototype that can be transferred to other complex network systems, such as shipping, air traffic, electricity transmission, health care systems, supply chain management, internet connectivity and finance.
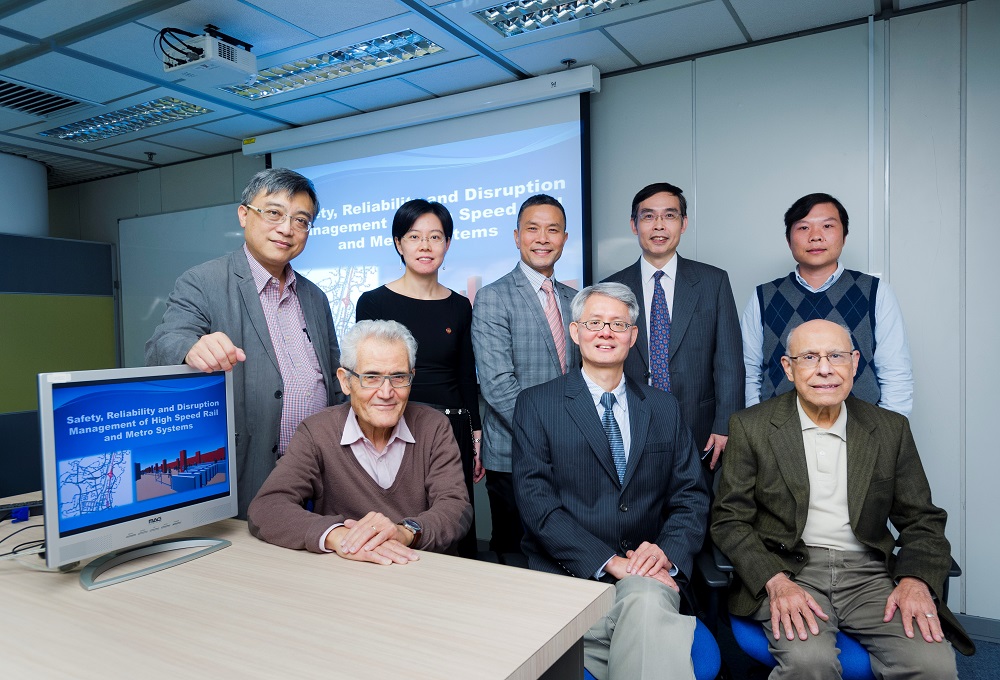
This research article originated from CityU RESEARCH.