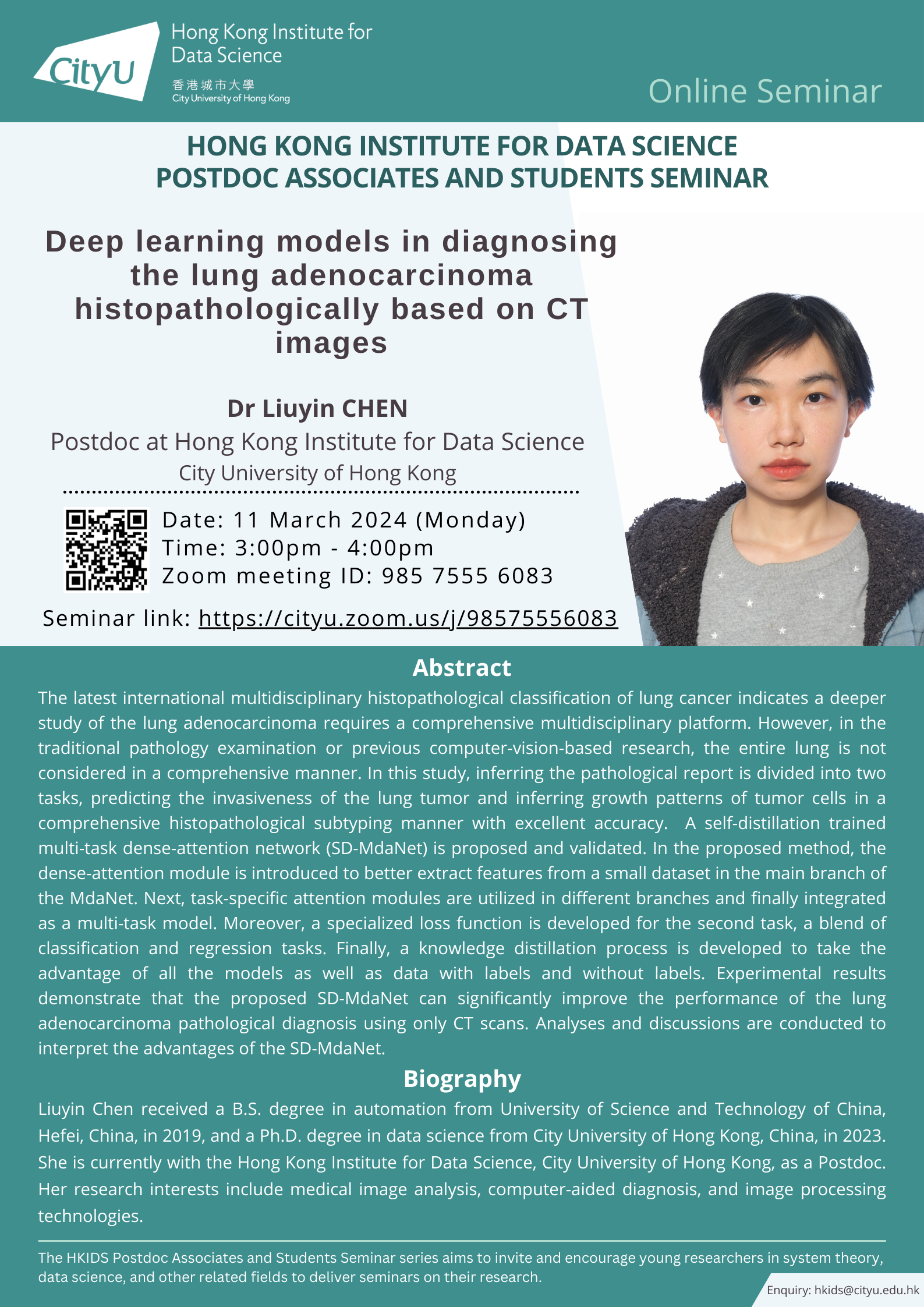
The latest international multidisciplinary histopathological classification of lung cancer indicates a deeper study of the lung adenocarcinoma requires a comprehensive multidisciplinary platform. However, in the traditional pathology examination or previous computer-vision-based research, the entire lung is not considered in a comprehensive manner. In this study, inferring the pathological report is divided into two tasks, predicting the invasiveness of the lung tumor and inferring growth patterns of tumor cells in a comprehensive histopathological subtyping manner with excellent accuracy. A self-distillation trained multi-task dense-attention network (SD-MdaNet) is proposed and validated. In the proposed method, the dense-attention module is introduced to better extract features from a small dataset in the main branch of the MdaNet. Next, task-specific attention modules are utilized in different branches and finally integrated as a multi-task model. Moreover, a specialized loss function is developed for the second task, a blend of classification and regression tasks. Finally, a knowledge distillation process is developed to take the advantage of all the models as well as data with labels and without labels. Experimental results demonstrate that the proposed SD-MdaNet can significantly improve the performance of the lung adenocarcinoma pathological diagnosis using only CT scans. Analyses and discussions are conducted to interpret the advantages of the SD-MdaNet.
Speaker: Dr Liuyin CHEN
Date: 11 March 2024 (Mon)
Time: 3:00pm - 04:00pm
Poster: Click Here
Latest Seminars
Biography
Liuyin Chen received a B.S. degree in automation from University of Science and Technology of China, Hefei, China, in 2019, and a Ph.D. degree in data science from City University of Hong Kong, China, in 2023. She is currently with the Hong Kong Institute for Data Science, City University of Hong Kong, as a Postdoc. Her research interests include medical image analysis, computer-aided diagnosis, and image processing technologies.