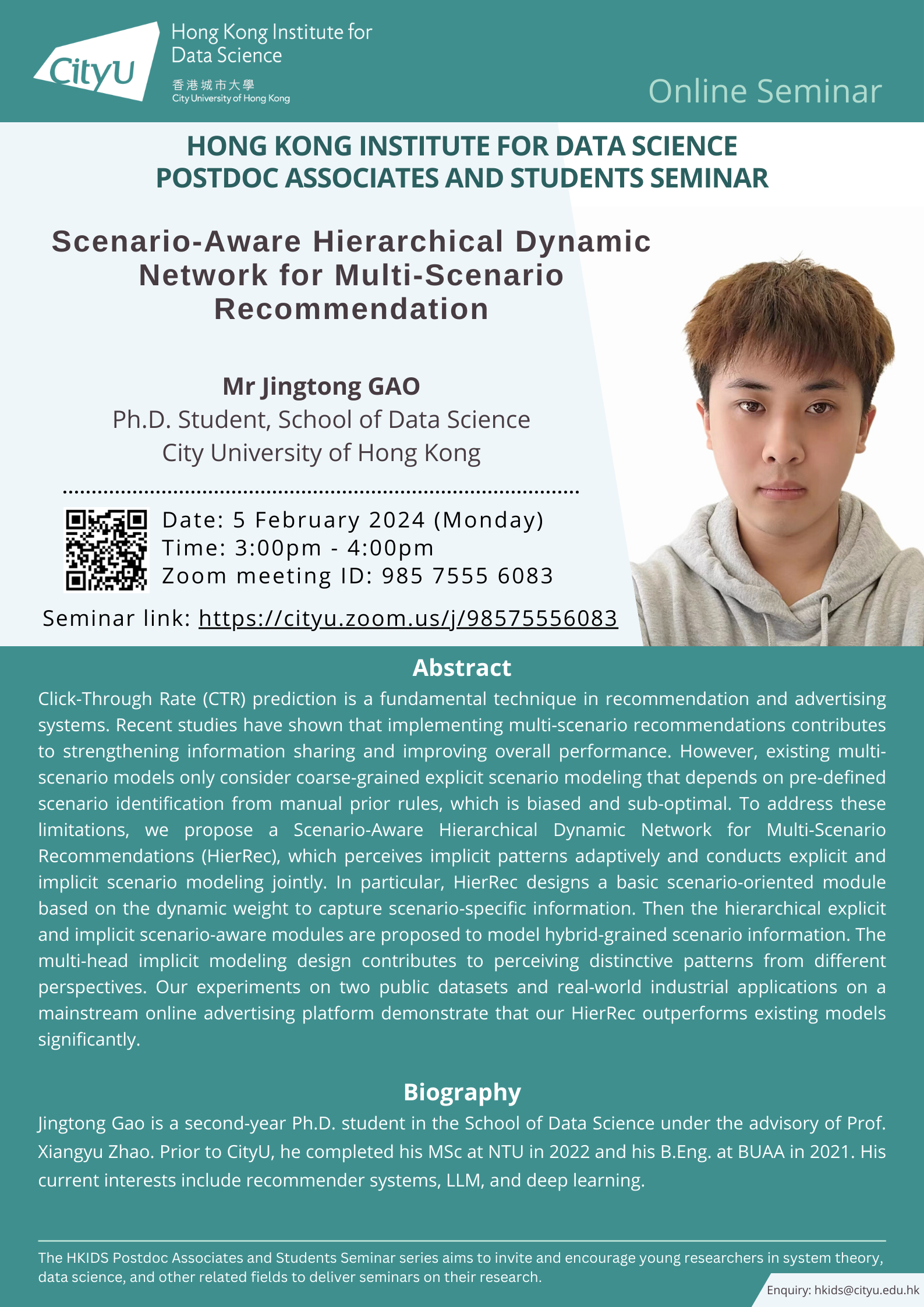
Click-Through Rate (CTR) prediction is a fundamental technique in recommendation and advertising systems. Recent studies have shown that implementing multi-scenario recommendations contributes to strengthening information sharing and improving overall performance. However, existing multi-scenario models only consider coarse-grained explicit scenario modeling that depends on pre-defined scenario identification from manual prior rules, which is biased and sub-optimal. To address these limitations, we propose a Scenario-Aware Hierarchical Dynamic Network for Multi-Scenario Recommendations (HierRec), which perceives implicit patterns adaptively and conducts explicit and implicit scenario modeling jointly. In particular, HierRec designs a basic scenario-oriented module based on the dynamic weight to capture scenario-specific information. Then the hierarchical explicit and implicit scenario-aware modules are proposed to model hybrid-grained scenario information. The multi-head implicit modeling design contributes to perceiving distinctive patterns from different perspectives. Our experiments on two public datasets and real-world industrial applications on a mainstream online advertising platform demonstrate that our HierRec outperforms existing models significantly.
Speaker: Mr Jingtong GAO
Date: 5 February 2024 (Mon)
Time: 3:00pm - 04:00pm
Poster: Click Here
Latest Seminars
Biography
Jingtong Gao is a second-year Ph.D. student in the School of Data Science under the advisory of Prof. Xiangyu Zhao. Prior to CityU, he completed his MSc at NTU in 2022 and his B.Eng. at BUAA in 2021. His current interests include recommender systems, LLM, and deep learning.