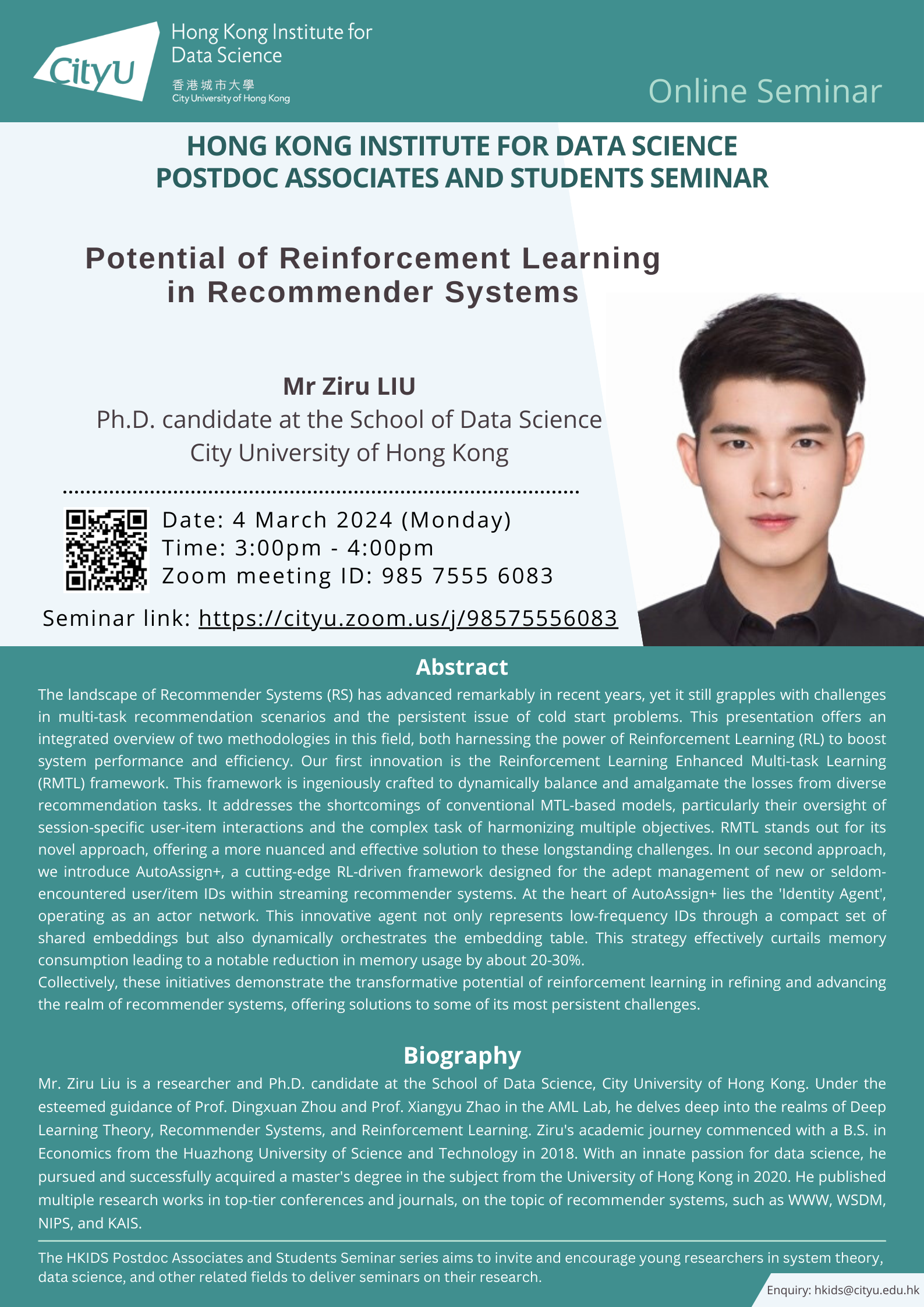
The landscape of Recommender Systems (RS) has advanced remarkably in recent years, yet it still grapples with challenges in multi-task recommendation scenarios and the persistent issue of cold start problems. This presentation offers an integrated overview of two methodologies in this field, both harnessing the power of Reinforcement Learning (RL) to boost system performance and efficiency. Our first innovation is the Reinforcement Learning Enhanced Multi-task Learning (RMTL) framework. This framework is ingeniously crafted to dynamically balance and amalgamate the losses from diverse recommendation tasks. It addresses the shortcomings of conventional MTL-based models, particularly their oversight of session-specific user-item interactions and the complex task of harmonizing multiple objectives. RMTL stands out for its novel approach, offering a more nuanced and effective solution to these longstanding challenges. In our second approach, we introduce AutoAssign+, a cutting-edge RL-driven framework designed for the adept management of new or seldom-encountered user/item IDs within streaming recommender systems. At the heart of AutoAssign+ lies the 'Identity Agent', operating as an actor network. This innovative agent not only represents low-frequency IDs through a compact set of shared embeddings but also dynamically orchestrates the embedding table. This strategy effectively curtails memory consumption leading to a notable reduction in memory usage by about 20-30%.
Collectively, these initiatives demonstrate the transformative potential of reinforcement learning in refining and advancing the realm of recommender systems, offering solutions to some of its most persistent challenges.
Speaker: Mr Ziru LIU
Date: 4 March 2024 (Mon)
Time: 3:00pm - 04:00pm
Poster: Click Here
Latest Seminars
Biography
Mr. Ziru Liu is a researcher and Ph.D. candidate at the School of Data Science, City University of Hong Kong. Under the esteemed guidance of Prof. Dingxuan Zhou and Prof. Xiangyu Zhao in the AML Lab, he delves deep into the realms of Deep Learning Theory, Recommender Systems, and Reinforcement Learning. Ziru's academic journey commenced with a B.S. in Economics from the Huazhong University of Science and Technology in 2018. With an innate passion for data science, he pursued and successfully acquired a master's degree in the subject from the University of Hong Kong in 2020. He published multiple research works in top-tier conferences and journals, on the topic of recommender systems, such as WWW, WSDM, NIPS, and KAIS.