
Opportunity
Prostate cancer is the second most common type of cancer in men. However, the two main methods of screening for prostate cancer in current clinical practice, namely prostate-specific antigen testing and digital rectal examination, have very limited diagnostic accuracy. They may even lead to over-diagnosis, i.e., the diagnosis of harmless and asymptomatic medical conditions, which can cause patients avoidable psychological stress and lead to unnecessary treatments. To screen for early-stage prostate disease and avoid unnecessary biopsies, artificial intelligence (AI) assisted diagnostic algorithms have been developed. These algorithms utilize magnetic resonance imaging to predict prostate health status based on, for example, the presence of clinically significant biomarkers. However, existing AI-assisted diagnostic algorithms for prostate cancer screening require clinicians to collect a large volume of data and provide detailed manual annotations, incurring high costs in terms of time and labor.
Technology
CityU researchers have devised a novel method of automatic prostate disease diagnosis based on magnetic resonance imaging (MRI). Their invention will help clinicians to predict the health status of a patient’s prostate more efficiently and reliably than can be achieved using existing MRI-based methods. Whereas most AI-assisted diagnostic algorithms have to be trained with detailed manual annotations of 3D MRI scans (e.g. pixel-wise, patch-wise, or slice-wise), the new approach requires only patient-level annotations, which are much more readily available. Specifically, the invention is termed the Graph-based Prostate Diagnosis Network (GPD-Net). Its diagnostic procedure has two stages: preliminary diagnosis and refined diagnosis. GPD-Net has been extensively experimentally tested and found to be superior in ease and diagnostic accuracy to existing MRI-based algorithms. In addition, the invention indicates the clinical significance of each slice in a prostate MRI scan. This allows clinicians to further confirm the predictions of GPD-Net without needing to laboriously view all of the slices in a 3D MRI scan.
Advantages
- Whereas existing algorithms need to be trained with large volumes of detailed manual annotations, the novel approach requires only patient-level annotations of prostate MRI scans, which are much more easily and quickly obtained. This reduces clinicians’ workload (time and labor).
- Significantly more accurate than existing diagnostic algorithms, as demonstrated in extensive experiments, thereby providing clinicians with more reliable and effective diagnostic assistance.
- Unlike existing methods, the invention also indicates the clinical significance of each slice in a prostate MRI scan. This enables clinicians to further confirm the algorithm’s predictions without needing to view all of the slices in the scan.
Applications
- Hospitals and other clinical settings—enabling clinicians to more accurately and reliably screen for prostate cancer while reducing their workload.
- Companies that develop medical equipment and medical diagnosis algorithms, especially those focusing on prostate screening.

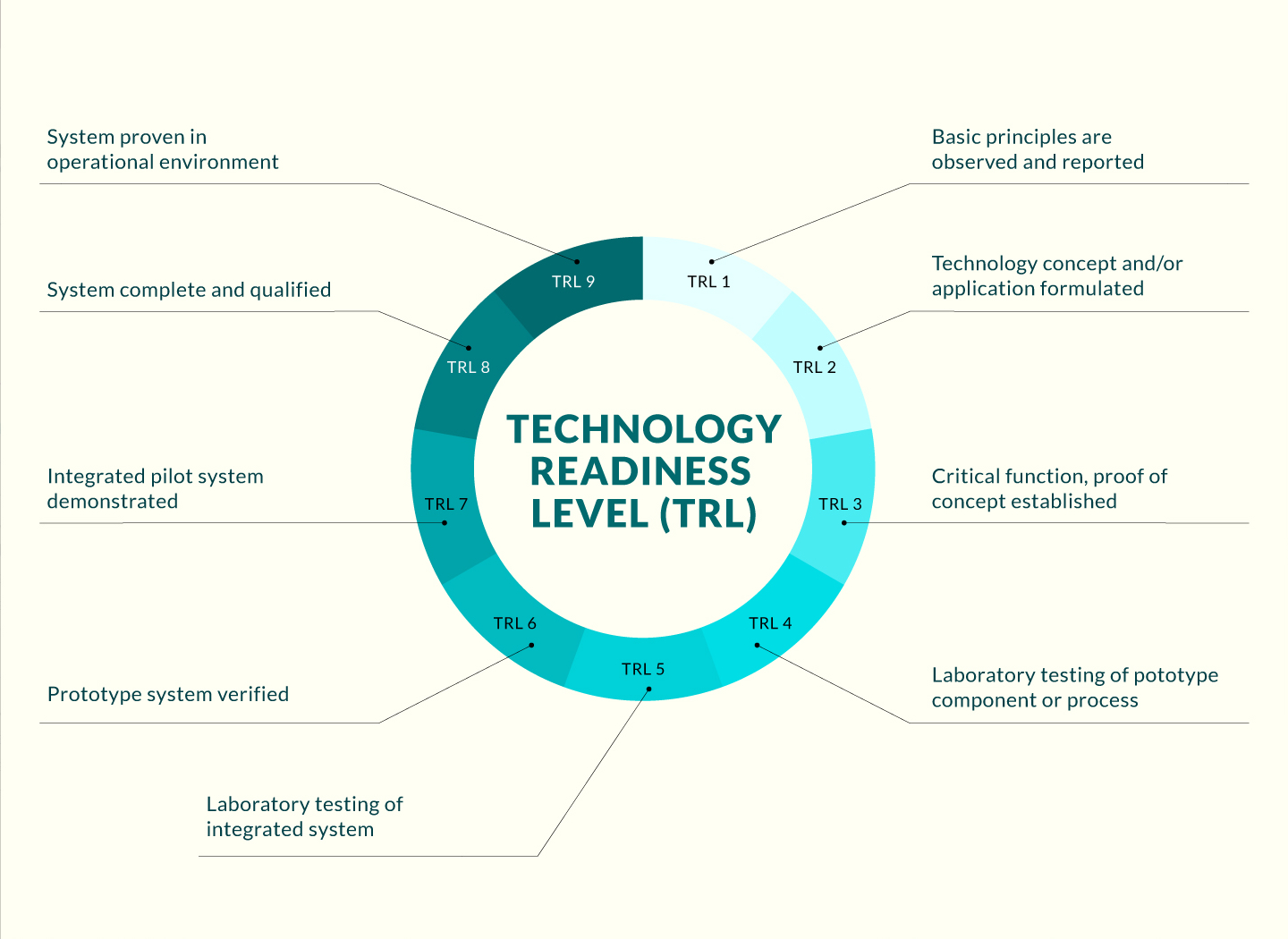
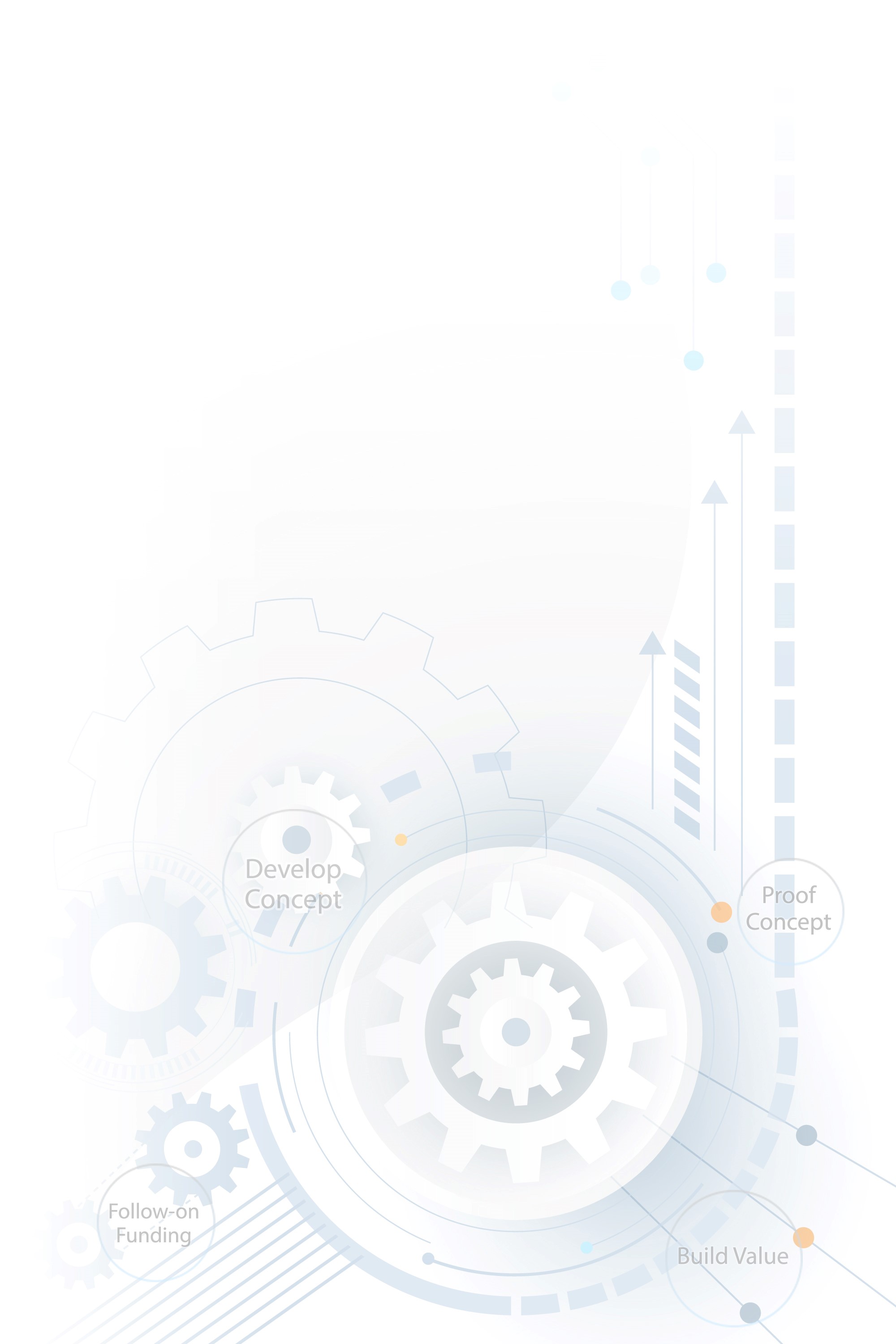