
Opportunity
The opportunity for this invention is significant for product based on or assisted by neural networks, such as self-driving car, video surveillance, intelligent loT devices and smart home business.
The global self-driving cars and trucks market size is expected to be approximately 6.7 thousand units in 2020 and is anticipated to expand at a CAGR of 63. l% from 2021 to 2030. The Video Analytics & Intelligent Video Surveillance Market reached USD 28.13 Billion in 2018 and is expected to attain a market value of USD 103.83 Billion by the end of 2027 by registering a CAGR of 15.14% across the globe.
The market size of Intelligent loT is 190 billion USD (2018) and that for Smart home was USD 79.90 billion in 2018 and is projected to reach USD 622.59 billion by 2026, exhibiting a CAG R of 29.3% during the forecast period.
Technology
Neural network compression and quantization are important tasks for fitting state-of-the-art models into the computational, memory and power constraints of mobile devices and embedded hardware. Recent approaches to model compression/quantization are based on reinforcement learning or search methods to quantize the neural network for a specific hardware platform. However, these methods require multiple runs to compress/quantize the same base neural network to different hardware setups.
In this invention, we propose a fully nested neural network (FN3) that runs only once to build a nested set of compressed/quantized models, which is optimal for different resource constraints. Specifically, we exploit the additive characteristic in different levels of building blocks in neural network and propose an ordered dropout (ODO) operation that ranks the building blocks. Given a trained FN3, a fast heuristic search algorithm is run offline to find the optimal removal of components to maximize the accuracy under different constraints. Empirical results validate strong practical performance of proposed approach.
Advantages
- Applicable to wide range of neural network components
- Better prediction accuracy
- More flexibility for deploying a neural network
Applications
- Autonomous Self Driving Vehicle
- Video Analytic
- Intelligent IoT
- Smart Home

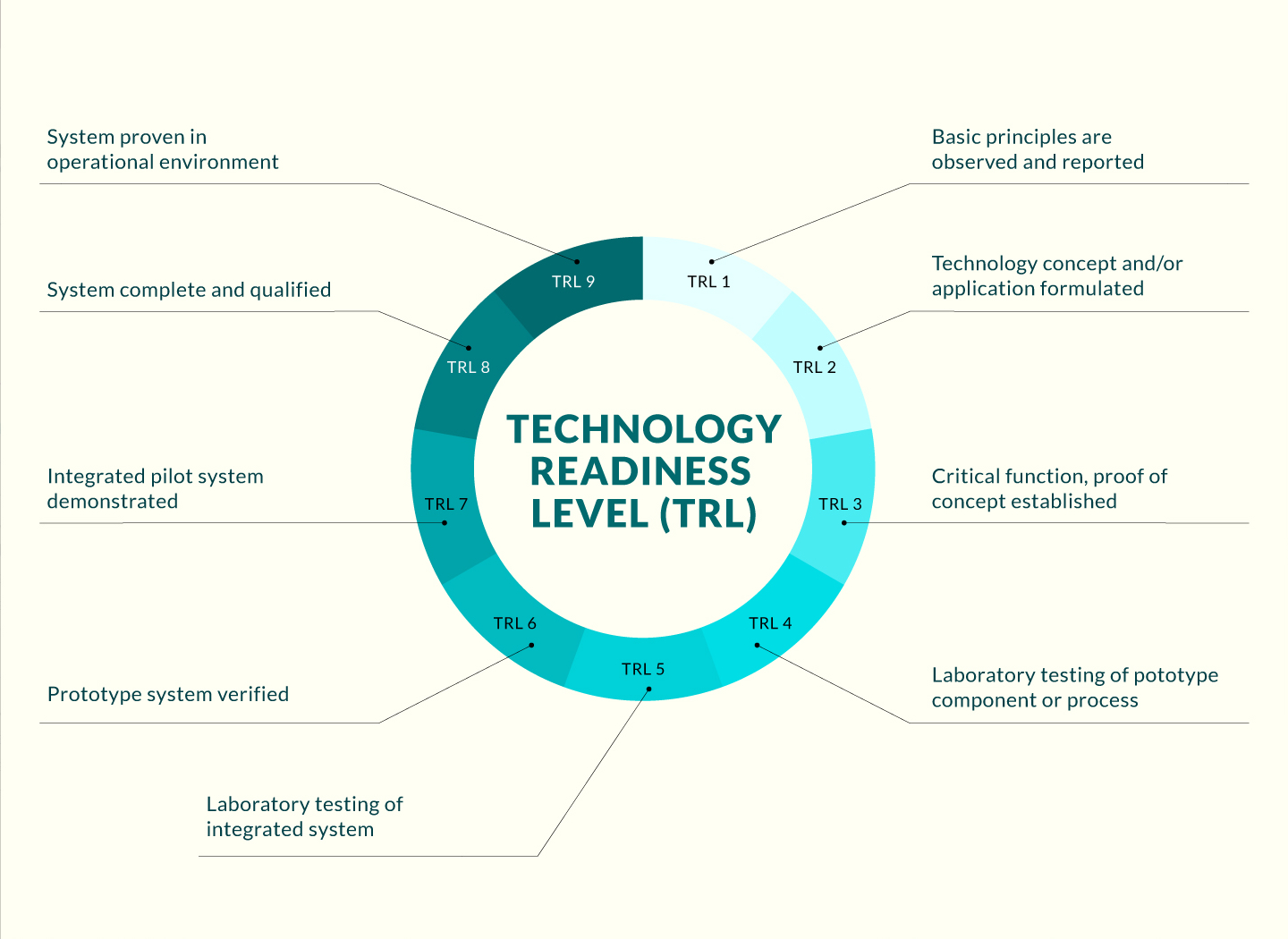
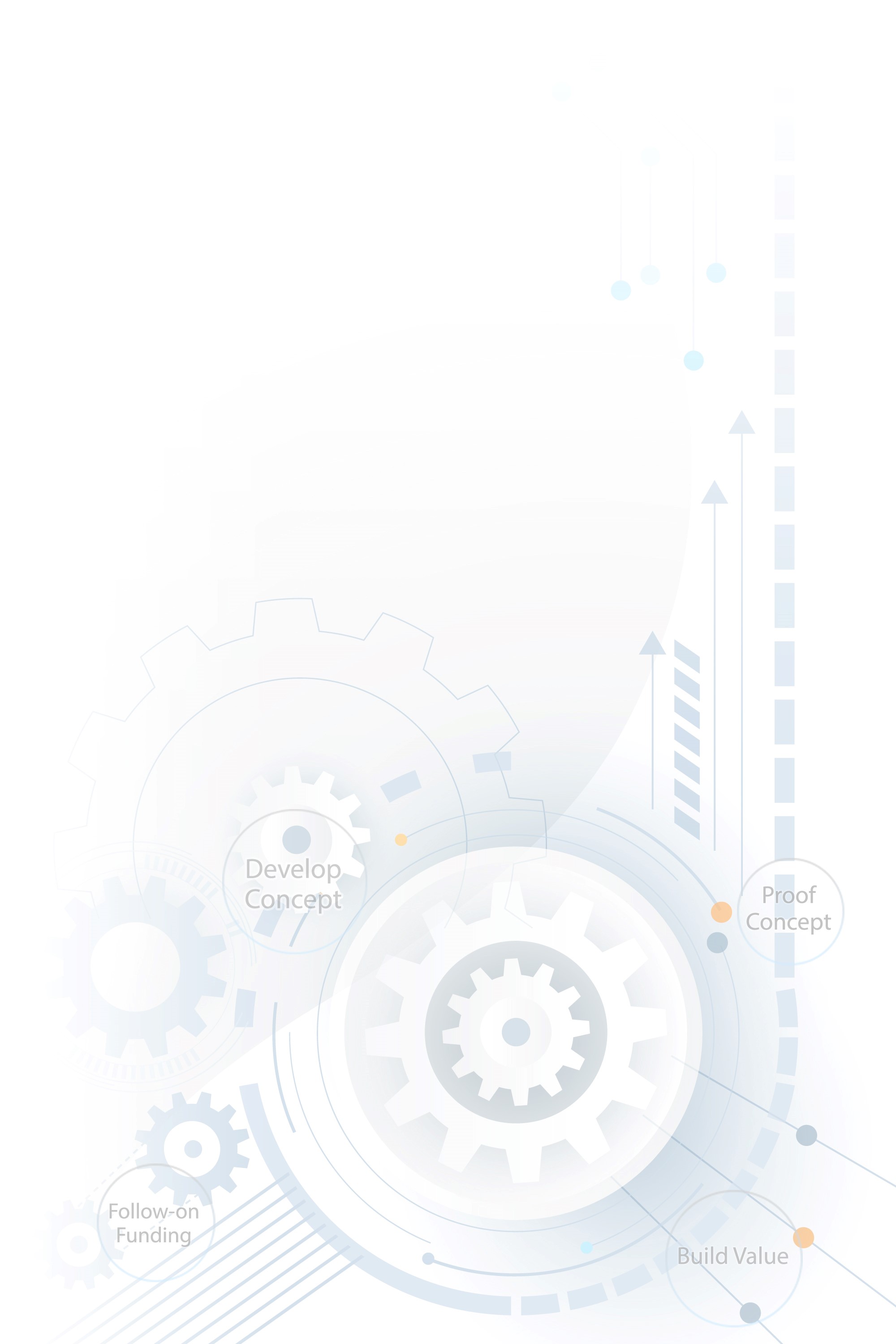