
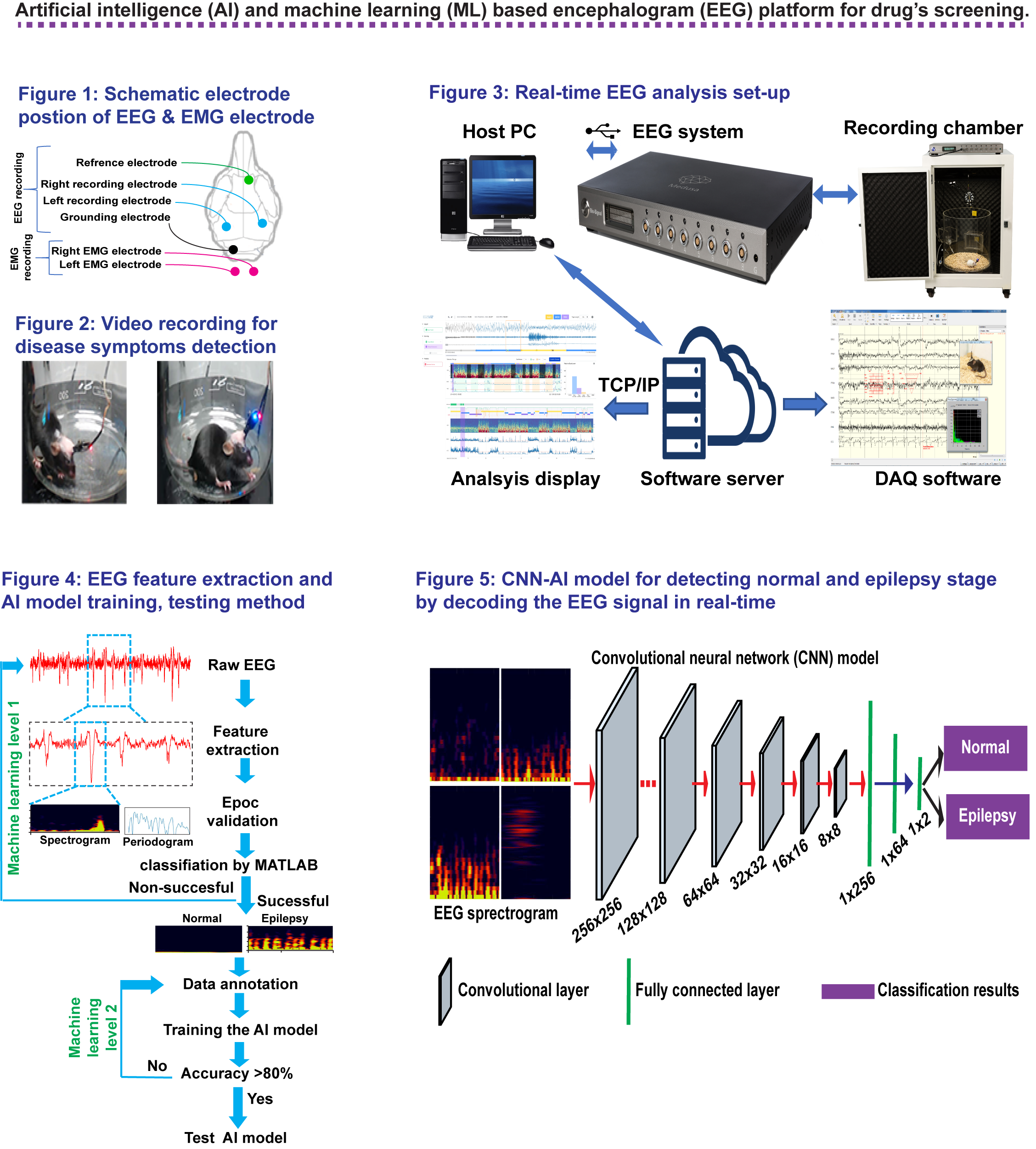
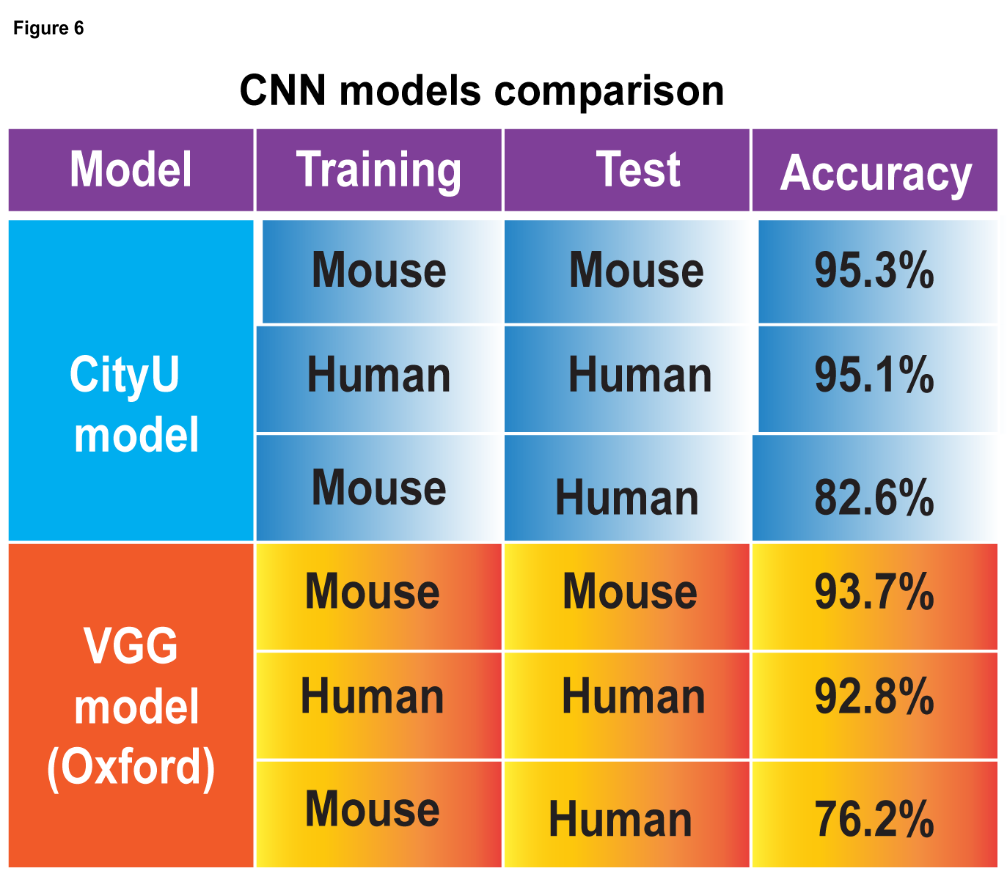
Opportunity
Preclinical studies are mandated by Food and Drug Administration (FDA, USA) and National Medical Products Administration (NMPA, China) before initiating first-in-human clinical trials. It offers dose range finding and repeated dose toxicity studies crucial for investigational new drug (IND) application. Preclinical studies are time consuming and require huge amount of investment. FDA requires toxicological studies in a minimum of two animal species before first in human (FIH) dosing, where large amount animals are involved, leading to increased cost and time for development of drug. Artificial intelligence (AI) and machine learning (ML) based encephalogram (EEG) study will improve the selection efficiency to narrow down the novel IND with maximum efficacy and thereby reduces the require time and investment. Further, AI and ML model will predict the outcome of human disease condition based on the rodent’s disease model. Therefore, the efficient EEG based AI and ML platform for potentially drug screening and diagnosis is highly demanded.
Technology
The inventors established EEG electrode implantation in mice model of epilepsy and Video-EEG were recorded. Seizure symptoms of epilepsy were evaluated using video recording and correlated with corresponding EEG activity through the developed Artificial Intelligence (AI) and Machine learning (ML) platform as preclinical study. Further, clinical (epileptic patents) EEG data were analysed using pre-clinical AI platform. Two level ML method has been followed for EEG features extractions. Spectrogram with defined time interval classified the EEG data into normal and epileptic. We achieved a accuracy of 93.7% in preclinical and 92.8% in clinical EEG data to predict the probability of normal or epilepsy phase. Our currently developed CNN model was compared with published AI model of VGG, Oxford. Overall, current model achieved high accuracy (82.6%) as compared with Oxford (76.2%).
Advantages
- AI platform will reduce initial screening within days and results will be available in real-time.
- The invented drug screening platform will narrow down the potent molecule and help to increase the change of successful clinical trial.
- In the following step of drug development, pharmaceutical company could omit investigating on hit and trial method.
- It will aid to exiting regulatory requirement of FDA or NMPA and fasten the process of drug discovery.
Applications
- Artificial intelligence (AI) and machine learning (ML) based encephalogram (EEG) platform could be applied to drug’s screening in real-time.
- The invented model predicts the translational probability of symptoms from pre-clinical to clinical disease condition.
2. 3rd Asia Exhibition of Innovations & Inventions Hong Kong (AEII) (2023) - Silver Award

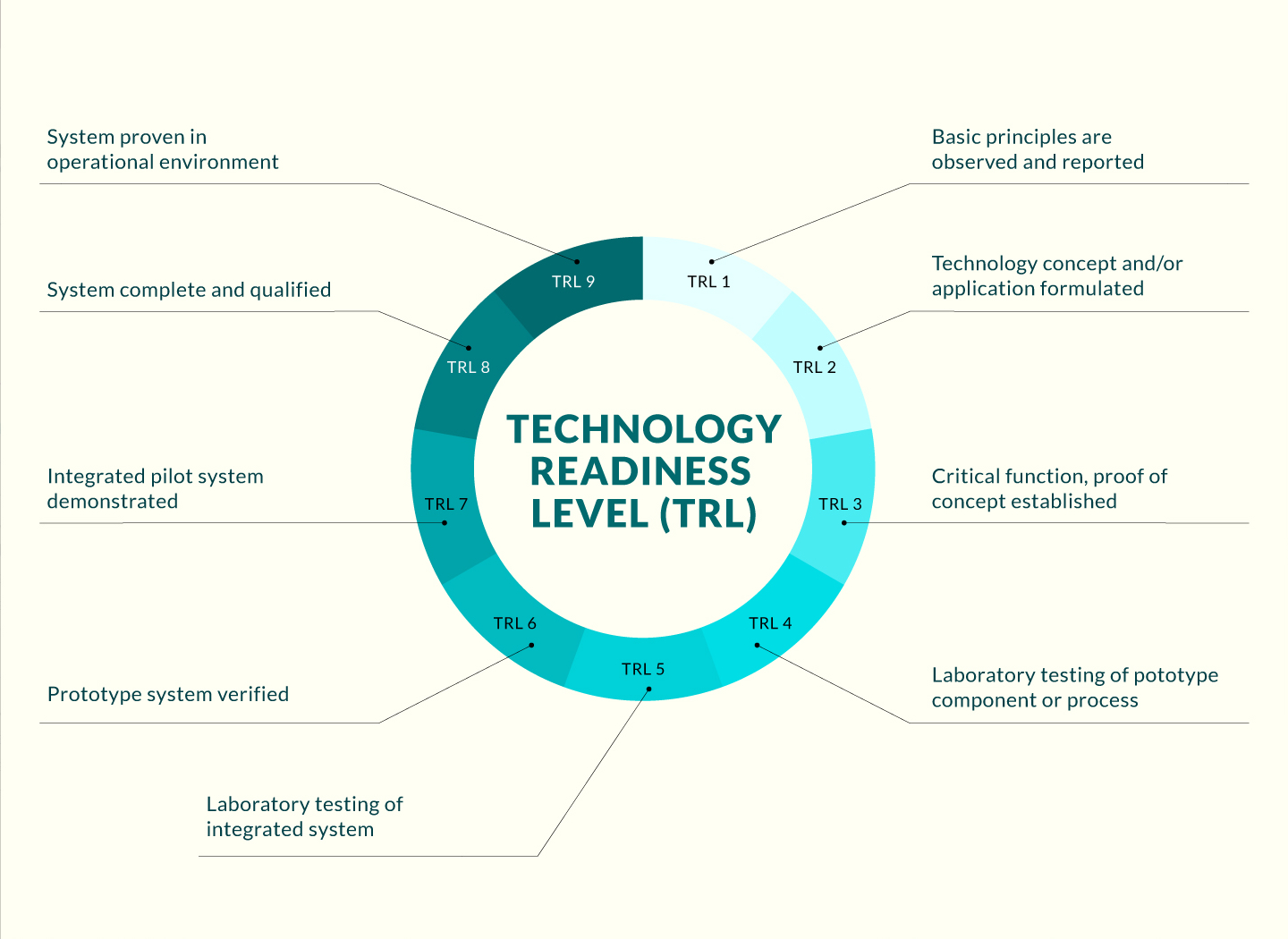
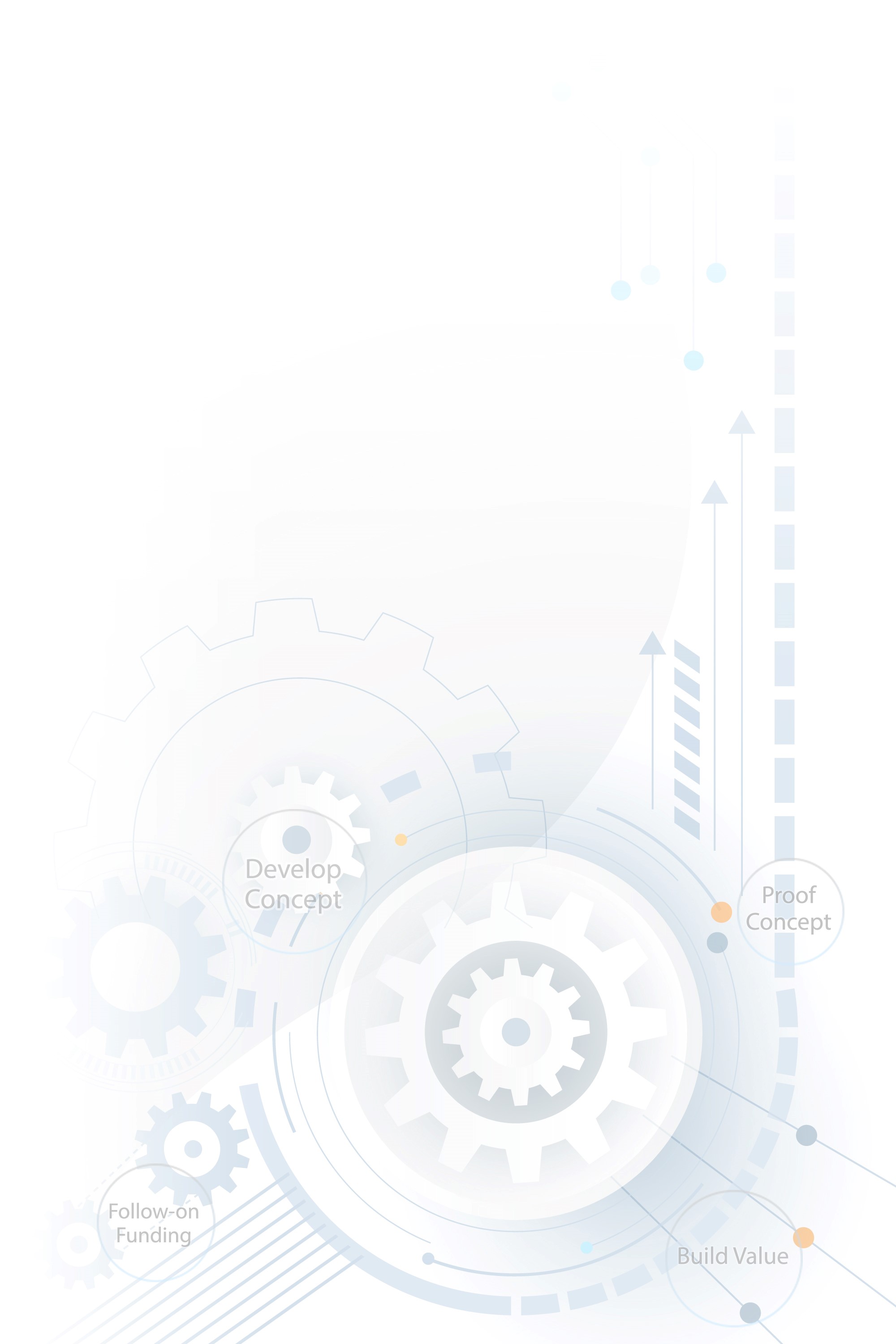